3D Medical Point Transformer: Introducing Convolution to Attention Networks for Medical Point Cloud Analysis
- Jianhui Yu1
- Chaoyi Zhang 1
- Heng Wang 1
- Dingxin Zhang 1
- Yang Song 2
- Tiange Xiang 1
- Dongnan Liu 1
- Weidong Cai 1
- 1University of Sydney
- 2University of New South Wales
Overview
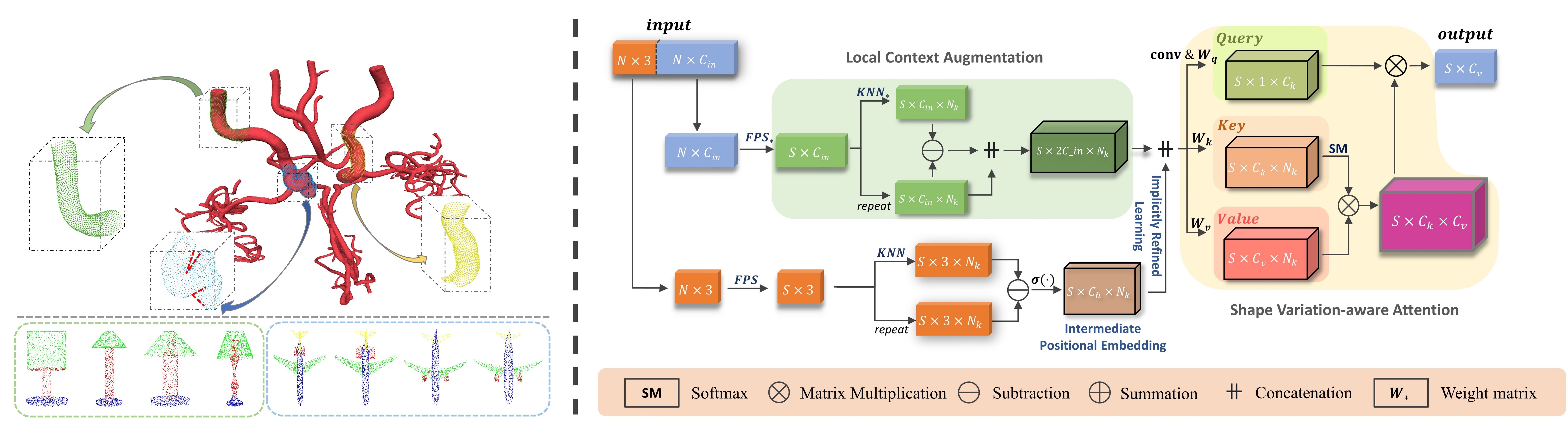
General point clouds have been increasingly investigated for different tasks, and recently Transformer-based networks are proposed for point cloud analysis. However, there are barely related works for medical point clouds, which are important for disease detection and treatment. In this work, we propose an attention-based model specifically for medical point clouds, namely 3D medical point Transformer (3DMedPT), to examine the complex biological structures. By augmenting contextual information and summarizing local responses at query, our attention module can capture both local context and global content feature interactions. However, the insufficient training samples of medical data may lead to poor feature learning, so we apply position embeddings to learn accurate local geometry and Multi-Graph Reasoning (MGR) to examine global knowledge propagation over channel graphs to enrich feature representations. Experiments conducted on IntrA dataset proves the superiority of 3DMedPT, where we achieve the best classification and segmentation results. Furthermore, the promising generalization ability of our method is validated on general 3D point cloud benchmarks: ModelNet40 and ShapeNetPart. Code is released.
Highlights
- State-of-the-art accuracy on IntrA classification: 0.936 (F1 score)
-
State-of-the-art accuracy on IntrA segmentation:
- IoU: 94.82% on healthy vessel and 82.39% on aneurysm
- DSC: 97.29% on healthy vessel and 89.71% on aneurysm
- ModelNet40 classification: 93.4%
- ShapeNet40 part segmentation: 84.3% (class mIoU)
BibTeX
If you find our project useful in your research, please cite:@article{yu20213d, title={3D Medical Point Transformer: Introducing Convolution to Attention Networks for Medical Point Cloud Analysis}, author={Yu, Jianhui and Zhang, Chaoyi and Wang, Heng and Zhang, Dingxin and Song, Yang and Xiang, Tiange and Liu, Dongnan and Cai, Weidong}, journal={arXiv preprint arXiv:2112.04863}, year={2021} }